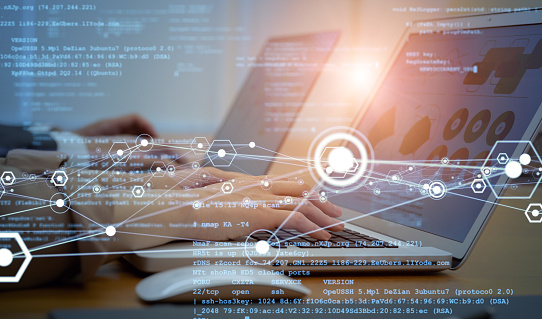
Your analysis and insights while dealing with data are only as good as the data you utilise. Your business cannot use data science analysis to make efficient and effective decisions if the data being analysed is unclean. Data cleaning is an essential component of data management that enables you to confirm that the quality of your data is high.
Data cleaning goes beyond only correcting grammatical or syntactic mistakes. It is a fundamental machine learning approach and a vital component of data science analytics. We will learn more about data cleansing today, including its advantages, potential drawbacks, and suggested learning strategies.
What is Data Science Cleaning?
The crucial process of correcting or deleting inaccurate, insufficient, or duplicate data from a dataset is known as data cleaning or data cleansing. Your workflow should start with data cleansing. It is very likely that you may duplicate or incorrectly classify data while dealing with massive datasets and merging several data sources. Your algorithms and results will lose trustworthiness if you have wrong or incomplete data.
Data cleaning is different from data transformation since it involves deleting data from your dataset that does not belong there. With data transformation, you alter the format or structure of your data. Data wrangling and data munging are two terms that are sometimes used to describe data transformation processes. We will concentrate on the data cleansing procedure today.
You can examine the aspects of the data to establish its quality and then balance them in light of the factors that are significant to your project and organisation.
When assessing your data, keep these five characteristics in mind:
Consistency: Do your data remain consistent between datasets?
Accuracy: Does your data closely reflect the actual values?
Completeness: Do your data contain all necessary details?
Validity: Does your data comply with any constraints or business rules?
Uniformity: Are the units of measurement used to specify your data consistently?
Step-by-step Process of Cleaning Messy Data
Delete any information that is not relevant.
You must first decide what analysis you will do and your downstream requirements. What inquiries or issues do you seek resolutions for?
Look carefully at your data to determine what is important and what you might not need. Remove information or observations that are not pertinent to your requirements later on.
If, for instance, you are analysing SUV owners and your data collection also includes information on sedan owners, this information is useless to your study and will simply bias your results.
If they are not a necessary component of your research, you should also think about deleting items like hashtags, URLs, emoticons, HTML tags, etc.
Remove duplicates from your data.
You will frequently get data duplicates if you are gathering data from several sources or departments, using scraped data for analysis, or receiving multiple surveys or customer replies.
Duplicate records increase storage needs and slow down analysis. But perhaps more significantly, if you train a machine learning model on a dataset that contains duplicate outcomes, the algorithm will probably give higher weight to the duplicates, depending on how frequently they have been copied. For well-balanced results, they must be eliminated.
Due to the ease with which AI systems can identify duplicate entries, even simple data cleaning technologies can be helpful in the deduplication of your data.
Solve any structural issues
Examples of structural faults are missing, inconsistent naming standards, erroneous capitalisation, misuse of certain words, etc. These can distort analyses because, even though they may be apparent to humans, most machine learning programs wouldn’t catch the errors.
For instance, you would need to standardise the title if you were analysing two separate data sets, one containing a column for “women” and the other for “female.” Similarly, it is necessary to standardise data such as dates, addresses, phone numbers, etc., so that computers can interpret them.
Deal with missing data
To find empty text boxes, missing cells, unanswered survey questions, etc., scan your data or put it via a cleaning application. This can be the result of inaccurate or missing data. You must decide whether everything associated with this missing data—a whole column or row, a complete survey, etc.—should be destroyed, individual cells should be manually inputted, or everything should be left as is.
The analysis you want to do and how you preprocess your data will determine the best course of action to handle missing data. Sometimes, you may even reorganise your data to ensure that your analysis is unaffected by the missing numbers.
Remove outliers from the data.
Outliers are data points that deviate significantly from the norm and may cause your research to be overly biased in one direction. For instance, if you are averaging a class’s test results and one kid does not answer any of the questions. In this situation, you ought to think about eliminating this data point. Developments might be “really” much closer to the average.
The final analysis need not be accurate merely because one figure is substantially smaller or greater than the other values you are evaluating. An outlier need not be taken into account just because it exists. You must consider the type of study you are performing and the impact that deleting or maintaining an outlier will have on your findings.
Verify your data.
The final step in data cleansing, data validation, verifies your data’s authenticity and confirms that it is accurate, consistent, and structured correctly for usage in subsequent steps.
- Are the data you have adequate for your needs?
- Is it consistently formatted in a style or dialect your analytic tools can understand?
- Before analysis, do your clean data instantly support or refute your theory?
- Verify that your data is consistently formatted and clean enough for your purposes. Ensure all relevant data items are cross-checked to ensure no errors or omissions.
You may use machine learning and artificial intelligence (AI) algorithms to check that your data is accurate and suitable for usage. Once you have followed the proper data cleaning procedures, you may automate the process using data wrangling methods and tools.
Data Cleaning Tips
Establish the proper procedure and follow it consistently.
Create a data cleaning method that works for your data, your requirements, and the analytical tools you will be using. Since this is an iterative process, you must adhere strictly to your predetermined stages and methods for all future data and analysis.
It is crucial to remember that, although time-consuming, data cleansing is essential for your downstream operations. If you do not start with clean data, your analysis will almost certainly yield “garbage results,” which you will regret.
Use tools
You may utilise various useful data cleaning technologies to aid the process, ranging from simple and accessible to sophisticated and enhanced machine learning. Find out which data cleaning technologies are ideal for you by doing some study.
There are excellent tools available for both coders and non-coders alike. You may construct models for your needs if you know how to code. Look for tools with effective user interfaces so you can quickly test your filters on various data samples and see their effects.
Pay attention to errors and track the source of unclean data.
Track and note typical problems and patterns in your data to determine the appropriate cleaning methods to apply to data from various sources. The integration of analytic tools you frequently use will save you a ton of time and make your data even cleaner.
Wrapping It Up…
It is evident that data cleansing is an important, though somewhat tedious, step in doing any type of data analysis. If you follow the following instructions, your data will be fully prepared for downstream procedures.
To get reliable, practical findings that you can act on immediately, keep your procedures constant, and don’t skimp on data cleansing.
You can contact us at SG Analytics– a data science consultancy firm, for all your data science consulting service requirements.